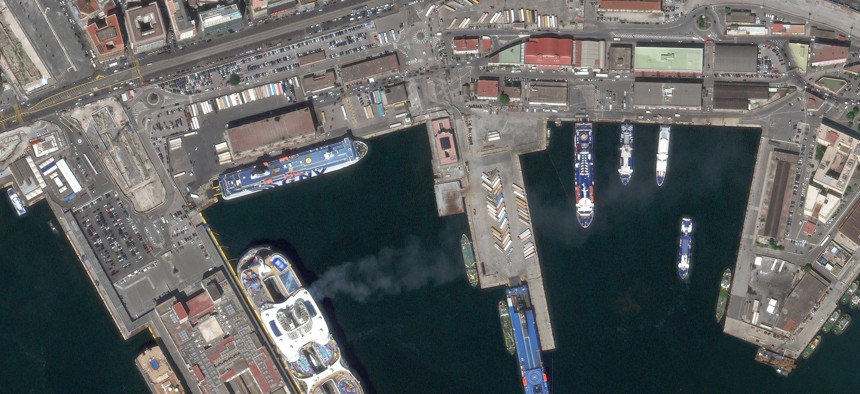
A satellite photo of the port of Naples, Italy. MAXAR
Who tells satellites where to take pictures? Increasingly, it’ll be robots, Maxar says
Automated scans of low-res imagery will cue high-res passes, while simulations will help manage ever-growing queues for service.
Maxar’s chief product officer Peter Wilczynski, who joined the space-imagery company over the summer, is spearheading an effort to build navigation systems that use 3D maps instead of GPS. But the Palantir alum is also working to develop systems to better manage the ever-growing queues for his company’s orbital-imagery services. He foresees automated tools that track changes captured by relatively low-resolution imagery to cue passes by Maxar's higher-resolution satellites.
This interview has been edited for length.
D1: What are some areas you want to explore, especially as the Defense Department looks to bring more commercial space companies into the fold?
Wilczynski: Our historical strength is really in foundational mapping. When we think about more operational missions, I think a lot about how we can…cut down the latency of our space-to-ground and ground-to-space communication. And as one of the only owner-operator-builder [satellite] companies in the world [that] actually designs, launches, and then owns and operates the satellite constellation, we have a lot of potential for integrating across the space and ground segments to really cut down the latency. And so that's a big focus for us.
So, for example, if you’re monitoring ships in the South China Sea, you’re trying to reduce the time needed to process and analyze that data?
I think that's exactly right. And, you know, I also think a lot about not just looking at the proximate analytic targets, so like a vessel that's moving, but actually, can you look backwards in the chain of events that would cause the vessel to move? So modeling site networks and understanding sort of how activity at one site could correlate with future activity at another site.
We're doing some work right now on monitoring mines. Those mines are going to affect the downstream supply chain. So, more activity there in a couple weeks means more activity here.
And so, as you think about managing a [satellite] constellation to do these tasks, as you get more supply on orbit, which I think we all know has happened in the last few years, you also get a lot more demand for that supply. The thing I'm excited about, from the Maxar perspective, is …experimenting with that supply-demand mapping for [with how] you take the different analytic admission requirements and then map them to the assets as they're continuously orbiting in space.
What are the biggest hurdles?
A lot of it actually is on the policy side. The core challenge of deconfliction and harmonization of requests. As you expand the number of entities and people who can make requests of a given constellation, you have to have some system of prioritizing those requests. And right now, a lot of that goes through a clearinghouse on the U.S. government side [that] has a pretty standardized prioritization scheme for deciding which requests turn into collections and taskings.
If you push for more real-time collection management, you have to have different, more sophisticated ways of intercepting and interrupting that existing priority queue to get something to the top. And so I think that constellation scheduling system—taking a request right now and actually putting it at the top of the queue, as opposed to putting it at the bottom of the queue because it came in later—that's really where the magic is going to happen.
We actually own our own constellation scheduler, we own the constellation, and we own a lot of the demand signals into that scheduler. And so I think there's a way where, without changing anything about what's on orbit today or the demand that's happening, we can actually experiment and play around with different ways of scheduling.
And so that's a place where we're doing a lot of sort of active development and, honestly, quite a bit of simulation. What are the different courses of action you could take? How do you actually simulate the orbitology, and then tie that to a schedule? So it's a place where I think there's actually a lot of potential to experiment in silico before shifting the whole collection-management system in practice to what's done in physical reality.
What does that experimentation look like?
Within the international business, we have a pretty well-established ladder system for prioritizing what we call satellite access windows. These are different windows of access to the satellites where customers are able to use the satellites and take pictures with them. So one of the experiments we're working on: is there an ability for a customer to basically jump ahead in line? And how would we actually balance that across all of our different customers?
I think what we're going to see in the U.S. government is something similar, where maybe different [combatant commands] actually start needing that same kind of adjudication process.
I hope to see this as a shift in the U.S. government, in general, towards more buying centers…more ability for different buyers to be creative about their requirements. And then, our ability to arbitrate between those buyers ultimately helps figure out the right mission solution for any given workflow.
What are you bringing to this job from your 12 years at Palantir?
They always talk about decisions, not data. And I think that is something that I'm bringing into the Maxar environment. Maxar is a company that makes a thousand decisions a day about where to take pictures. And I think what I saw is that when you apply better data and better algorithms to those core decisions that you're making as a business, you can actually improve the decision making pretty rapidly.
When I think about the products that we offer, whether it's tasking products, data products, analytics products, all of them fundamentally point back to “Where are we pointing the satellites every minute of every day?” And so I think that focus on decision-making, and really decision-making loops, is something that I definitely carry forward.
A lot of the geospatial industry, I think, has been really focused on [tasking] a satellite, [getting] an image, [and doing] analytics on that image. That's been the flow of what people think about as what we're trying to provide as an industry, whether in the commercial or government sector. I'm really focused on once you have the analytic and you have the insights out of that [machine learning] algorithm, how do you actually tie that back to tasking? Where do you task next? And applying that whole idea of loops to the actual work products we're producing, so that you can move towards a world of more autonomous tasking, where humans and analysts don't necessarily need to be manually looking at an image. But the images themselves can be driving more tasking, and can be sort of intelligently collecting data without a really manual collection management process happening.
How does that work?
We have some work going on right now that's doing this, at maybe a lower frequency. There’s Sentinel data, which is 10-meter data that's collected pretty regularly of the whole globe. You can run a machine-learning algorithm on that data that tells you where might have buildings and roads be being constructed. It's not going to give you the level of detail you need to turn that into a map, but what it can do is it can say, “Hey, at low resolution, we see change here. Why don't we take an image of that change so that we can digitize it at high resolution?” So you're [getting] output of a machine-learning algorithm applied to really low-resolution imagery, putting that as an input to your tasking, and then tasking high-resolution imagery to collect that region and validate whether or not the machine-learning algorithm was correct or wrong about the building that’s been constructed.
So grainy photos taken every day with automatic detection of changes that triggers a satellite to take a better picture of that change.
Exactly. So like, you could imagine taking a daily picture at low resolution and sort of saying, "OK, you know, the change started; the change ended." And that's probably going to be durable for another few years, right? They're not going to knock the building down immediately, but if you try to take that high-resolution picture every day, that asset can be a lot more expensive than the low-resolution asset for that particular mission.
I would imagine it's better than an analyst having an alert to remind them to check an area to see if something has changed.
I think about it a lot. If you walked into Wall Street in the 1980s, you would have a bunch of people yelling about which stocks to buy and which bonds to buy and which options to buy. You walk in now and you hear a bunch of humming computers. There's still people programming those algorithms figuring out what trades to make. They're just not manually making the trades. The trades are being made automatically with a machine-to-machine system that is able to, in a much more sophisticated way, set the right price for whatever instrument people are trying to buy. And so I think we've seen this transition in a lot of other industries, whether it's online advertisement, or taxi dispatching, or trading, and I think it's something that I'm excited about. We're finally at the point where we have enough supply, we have enough demand that really the market making is where a lot of value needs to be developed in the next few years.
What products and services will come in 2025 or early 2026?
There's really two major pushes that I'm focused on right now. One is sort of this broader evolution within cartography, and especially digital cartography, from sort-of 2D representations of the world to 3D representations of the world and then to vector representations of the world.
When you think about your experience using Google Maps or Apple Maps as a consumer, if you're anything like me, you often use the vector-based navigation system. You don't look at the satellite imagery. You look at the buildings and the roads and the schools and the Starbucks that exist on the map that have been extracted from that satellite imagery. And so one of the big threads is pushing from that raw data into the derived data. That takes a lot of work, a lot of machine learning, a lot of training to get to, but ultimately is a much more semantically meaningful representation of the world that really helps users understand, not just navigating between two points, but between two places.
The other push is really sort of moving up the stack and up the value chain. From tasking to data into actual end-to-end integrated solutions. We have the world's biggest 3D representation of the globe at Maxar. We put that together by sort of processing the raw 2D imagery into sort of a 3D globe. And we're thinking a lot and working a lot with our customers on how [to] help them use that.