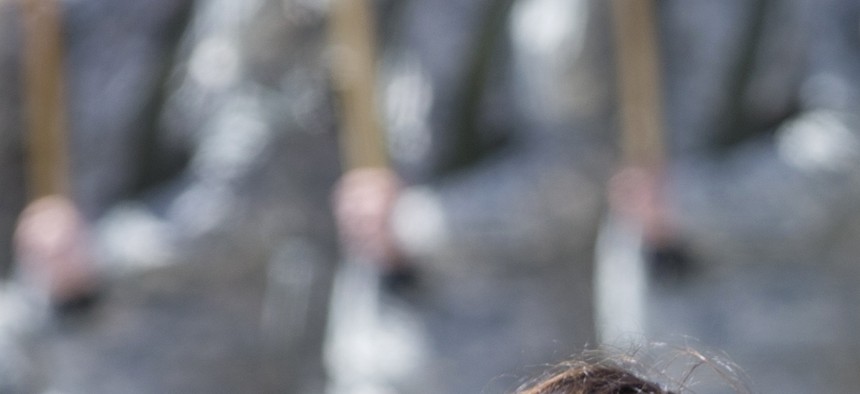
President Barack Obama and First Lady Michelle Obama pay their respects during a memorial ceremony at Fort Hood on Wednesday. AP Photo/Carolyn Kaster
Could Big Data Have Prevented the Fort Hood Shooting?
Researchers say an experimental software program might have been able to get Army Spec. Ivan Lopez help before he pulled the trigger. Here’s how. By Patrick Tucker
The federal government stopped funding a medical data screening program last year that researchers say might have prevented the Fort Hood shooting.
Had Army Spec. Ivan Lopez been enrolled in the Durkheim Program, which uses an algorithm that mines social media posts for indicators of suicidal behavior, it might have picked up clues that a clinician could have missed in time for an intervention.
“Given the highly agitated state of the shooter, we may have been able to get him help before acted, had he been in our system,” said Chris Poulin, one of the founders of the Durkheim Project, which received $1.8 million from the Defense Department’s Defense Advanced Research Project Agency, or DARPA, in 2011 until funding was halted in 2013.
DARPA’s funding for projects like Durkheim and other cutting-edge research and innovation is finite in scope and is meant to push state-of-the-art projects forward. Projects typically last for 3 to 5 years, but the scope of money available for researchers can change depending on agency objectives, available funds and other factors. “It is a telling illustration that we don't have any DOD funding at the present time despite being a 'successful' DARPA project,” Poulin said, especially given the growing problem of suicide among veterans. The Department of Veterans Affairs estimates that 22 veterans kill themselves every day.
DARPA declined a request for comment.
Last week, Lopez shot and killed three fellow soldiers and wounded 16 others, before turning the gun on himself. While Army officials said Lopez was being treated for mental health issues, he wasn’t considered suicidal. Still, Poulin said monitoring his Facebook, Twitter and Linkedin profiles might have provided some warning signs.
While Poulin and his fellow researchers have not yet built extensive models to predict 'harm of others,’ based on text clues the same tools could have been applied to Lopez and others, including former Army Maj. Nadal Hasan, who shot and killed 13 people at Fort Hood in 2009. While each soldier’s motivation and the circumstances surrounding his crime appear to be very different, both incidents may have been prevented using big data, Poulin said.
Hasan was driven by ideology and was not outwardly suicidal. Before he opened fire, Hasan openly opposed U.S. military policy in the Middle East, even writing about aspects of his intent and praising suicide bombers online. Lopez had sought treatment for mental health issues, including anxiety. Yet Lopez had no outward behavioral issues and showed no outward signs of violence or suicidal intention, according to officials.
Both tragedies represent the same sort of problem, however -- finding and processing predictive signals in available data, whether those signals are overt or deeply hidden. In a paper recently published in the journal PLOS ONE, Poulin shows how suicidal (and perhaps homicidal) intent can be predicted on the basis of language, even when suicide isn’t directly mentioned.
Poulin and his fellow researchers looked at Department of Veterans Affairs medical records for 70 soldiers who had committed suicide, 70 soldiers who had sought psychological help for something unrelated to suicide, and 70 soldiers who had not sought psychological help, but had come in for some other problem like a stubbed toe. Poulin and his colleagues converted the records into a data set and ran the set through a set of algorithms on a supercomputer to determine which words were more likely to be associated with which type of patient, called “bag of words” modeling. It “uses the frequency of words in a patient's medical report and completely disregards the linguistic structure, punctuation and structural markup of the original text,” the researchers said. “The records are not spell-checked or stemmed (i.e. reducing derivatives of words to their stem), and can include typographical errors and abbreviations of hospitals, clinics, departments, tests, procedures and orders.”
One of the things the researchers found was that words like “crying,” “preoccupied” and “splitting” (as in a divorce or breakup) were less characteristic of the suicide group than the group that had merely sought mental health. Whereas, words like “worthlessness” and “frightening” were more closely associated with individuals who had taken their own life. These distinctions, perhaps too small for individuals to notice, can make a big difference in diagnosis. Poulin and his fellow researchers have found that their algorithm accurately predicted suicide 65 percent of the time, a significant improvement over the average clinical diagnosis, which is about 50 percent. The algorithm has since been modified and can now predict 70 percent of the time, he said.
“An untrained clinician tends to be 50 percent [accurate] because of the subtlety of suicide [indicators,]” Poulin said. “A trained clinician who has read the right papers is still only getting one to two percent better than chance. There’s signal in terms of what meds [the subject] is on, demographics, that sort of thing. But there’s not much.”
The Durkheim Project, which entered its second phase last summer, is now being self-funded. But just 100 veteran and active duty volunteers are participating in the project. “We have the capability to monitor literally over 100,000 individuals,” Poulin said. “You have to monitor that many individuals to see a significant risk of suicide.”
Losing Defense Department backing has frustrated Poulin, and his experience was not isolated. The military, while significantly increasing funding and attention to suicide prevention programs, has given inconsistent attention to big data initiatives to detect suicide. Consider a separate 2012 project called the Prediction of Suicide and Intervention that was announced but was never fully launched. Poulin said these suicide prediction initiatives can be useful for predicting when soldiers might turn violent against their own kind.
“Scientifically you don’t have a problem delineating the rhetoric of self-harm versus the rhetoric of harm of others. In fact, on the highest level, it’s easier to see the rhetoric of harm of others.” For instance, Poulin said, disturbed individuals who are trending toward violence are more likely to become “verbose” in their communications. Hasan provides a case in point. As he veered closer to committing murder, he created more and more useful data indicating this intention.
What the algorithm can’t do is prescribe what sort of response is appropriate, or when. At what point does an intervention opportunity turn into prosecution of thought crime? Technologically, the problem is actually somewhat solvable, but there’s the challenge of false positives, selecting the wrong individual for screening or arrest on the basis of faulty inference. But if more data reduces the chance for false positives, does that justify more data collection, whether voluntary or involuntary?
Recent NSA disclosures have a created a public backlash against data surveillance. But the Fort Hood shooting has prompted calls for better invention and raised the question of why couldn’t we have done more?
“The technology exists,” to predict future events, Poulin said, “but we haven’t worked out the civil liberties and the policing procedures to use predictive analytics effectively.”