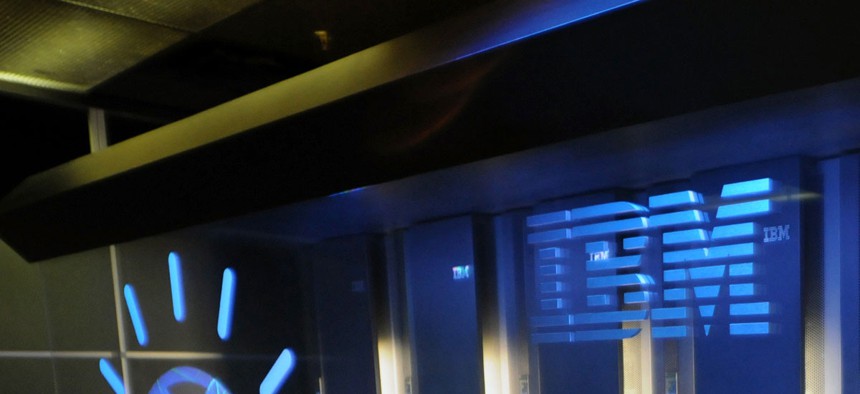
IBM's "Watson" computer at its offices in Yorktown Heights, N.Y. IBM, Bob Goldberg/AP
Teaching Machines How to Spell Will Help Catch Terrorists
It’s time anti-terrorism technology move beyond finding ways to replace humans and start finding ways to work with us. By David Murgatroyd
For all of our post-9/11 security , here’s a chilling fact: Boston Marathon bomber Tamerlan Tsarnaev might have been caught before his attack if the keepers of one terrorism watch list had done just one thing differently: spelled his name right.
A recent report from the House Homeland Security Committee exposed an embarrassing missed opportunity. Although Tsarnaev was on a watch list, he was not detained on his return to the United States from Dagestan because the list used a different spelling of his name: “Tsarnayev”. A human would have caught the error. But the growing number of requests like this that security professionals face means that we have to rely more and more on software. More and more, machines are the first guard on watch.
Obviously we need technology to be more robust to catch simple spelling variations in suspects’ names -- but how? Teaching software to understand the nuances of names and speech is an enormous national security challenge. If computer programs blindly start hunting misspellings without nuance, your name may be just a typo or two away from one of the hundreds of thousands of suspects on government watch lists. False positives erode public faith in security procedures, inconvenience innocent passengers and make it harder for security professionals to spot real bad actors.
Unfortunately, because the system Tsarnaev slipped through is classified, we can’t prescribe how to fix it. But we can learn from Jeopardy! When IBM’s computer “Watson” famously won the TV game show, it had access to 200 million pages of content via 90 powerful servers. But Watson made its own embarrassing mistake on a question in the category of “U.S. Cities.” The answer was: “Its largest airport is named for a World War II hero; its second largest, for a World War II battle.” Watson responded with the question, “What is Toronto?????”, which isn’t just the wrong answer, it’s in the wrong category! If only it had known Toronto is not a U.S. city it could have given the next answer down its list, the right one Chicago.
Why did this prove to be such a challenge? IBM explained in a blog post: “This is just one of those situations that’s a snap for a reasonably knowledgeable human but a true brain teaser for the machine.” First, there’s the variety and volume of data that Watson ingested. To Watson, “Toronto” is ambiguous. It had read about many places around the world called Toronto, including several towns in the Midwest. Second, modern artificial intelligence systems “think” about this data often by using fuzzy connections among strings of text. This could cause Watson to conclude that, because Toronto’s professional baseball team is in the American League, then Toronto itself is in the U.S.
When we move beyond game shows to stopping terrorists, curious errors can have fatal consequences. Such pressing challenges have grown text analytics technology into a $1 billion market with 25 percent additional growth predicted in 2015. While commercial organizations deploy text analytics to improve search results or provide a better shopping experience, government leaders seek to enhance military and intelligence operations and improve public-safety. But to truly measure the return on this investment requires not just measuring how systems help users, but also determining how users help systems – and thereby crossing technology’s next frontier.
Watson represents a pinnacle of the era of automating human tasks – the culmination of over a half-century of technology advancements. But as the Toronto example makes clear, Watson needs a partner. Assisting humans in their tasks is the theme of the next half-century. Just imagine if Watson collaborated with Jeopardy! champion Ken Jennings, or just with you or me. We could establish a virtuous cycle where our correction of simple errors would result in subtle improvements down the line.
This sort of collaboration is available now. If an agent at JFK airport the day Tamerlan Tsaernaev came through had had a newer system that handled more spelling variation, she would have seen the “Tsaernayev” match but also some innocent results. She would have eliminated these mismatches by diving deeper into the person’s itinerary or history on the watch list. Every time she interacts with the data – honing in on people of interest and discarding the misfires – the computer understands this and presents the next batch of results with greater awareness of what is being sought. All of this is conducted in a very natural way, much like two people working together, side-by-side.
As the collaboration between the person and the computer becomes richer, each will want the ability to communicate their confidence about certain judgments to the other. You can see a hint of this in the question marks in Watson’s “What is Toronto?????”. It’s almost as if it was asking for help. Those question marks would be a strong clue to a human collaborator to focus on that answer and its alternatives.
Confidence can also avoid problems when the system’s default settings filter out either too much or too little. When U.S. officials are planning for a future event, like a visiting head of state, and need to assess the security risk, they want the deepest possible information on potential threats and bad actors. In this case, because they have the time, analysts will want to investigate even items of low confidence that might point to a security threat. However, when responding to a time sensitive event, such as after the Boston bombing, officials only have time to pay attention to the highest quality results so they can filter out results with no less than 90 percent confidence. The remaining results of less than 90 percent confidence will be available later, when they can conduct deeper inquiry and analysis.
Even with a collaborative interface between the human and computer that allows the expression of confidence, if the computer is stuck thinking about a string of texts then the user may have a hard time feeding it useful information. Telling Watson that “Toronto” is a wrong answer to the question above will only help if it has some way of internalizing that the most popular real-world city by that name is not in the U.S.
Historically, national security operatives and analysts have attempted to overcome computers’ focus on strings of text by tediously curating complex search queries, creating long queries of keywords, joined by ANDs, ORs and NOTs to try to capture every possible string of relevant text. Unfortunately this method fails at finding new variations, misspellings or terms. Instead, analysts should be able to issue queries with respect to known key players – persons, places and other things – in the data. The system can filter the data based on real-world things and the analyst can refine the results.
In addition, a system centered on key players instead of keywords can help users track “ghosts” – entities appearing in raw data but that are otherwise unknown. Imagine a hypothetical terrorist incident, where a few hours earlier the computer sees social media messages on networks of interests. By focusing on key players, not keywords, it realizes that although the level of “chatter” is normal, a single person is being frequently mentioned in these messages by a variety of names. Although this person is unknown to the system, it’s able to connect all the names together as referring to him and to flag him as receiving an unusually high amount of attention. An analyst is alerted to this, reviews the messages that the computer has grouped and takes action.
It’s an exciting time to be applying human-computer collaboration to the challenges before us. Systems like Watson may need us as much as we need them.
David Murgatroyd is vice president of engineering at Basis Technology. He has been building human language technology systems since 1998. Twitter: @dmurga