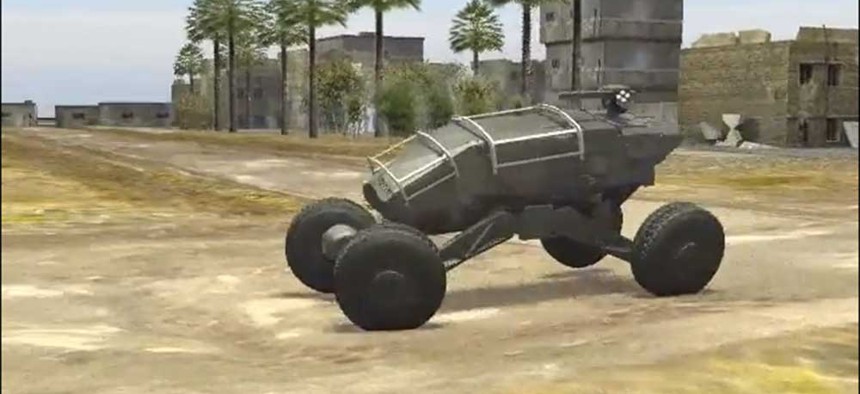
A screenshot of the Ground X Vehicle technology maneuvering. DARPA
The Military Wants a Vehicle That Can Dodge Rockets By Itself
The military wants to build future vehicles that don’t just withstand assaults but avoid them. By Patrick Tucker
The year is 2020; the setting, a battlefield in the Middle East. An armored Army vehicle bounds over low dunes on its way to a checkpoint when a local tribal leader fires a shoulder-mounted missile directly at the fast-moving truck. The targeting is dead on and the missile is moving too fast for the human driver to take evasive action. But the vehicle itself detects the vibrations of rocket in motion via an array of advanced sensors. Acting at the speed of electric current, the vehicle’s raised-wheel axis extends out beneath it, dropping it several feet, like a newborn falling on shaky legs. The rocket glides over the top of the vehicle missing it. The result? No casualties to report.
The above scenario is what the Defense Advanced Projects Research agency has in mind with its Ground X-Vehicle Technology (GXV-T) program . The cost of vehicle armor going up and its effectiveness going down, the military wants to build future vehicles that don’t just withstand assaults but predict and avoid them.
The goal of the program is to build vehicles that weigh half as much as those of today, require half the crew, move twice as fast and can access 95 percent of the sorts of terrains that the military might encounter. The agency plans to award contracts by April of next year, which will kick off two years of funded research.
“Inspired by how X-plane programs have improved aircraft capabilities over the past 60 years, we plan to pursue groundbreaking fundamental research and development to help make future armored fighting vehicles significantly more mobile, effective, safe and affordable,” program manager Kevin Massey said in a press release.
On Friday, the agency released a concept video to illustrate what that means. The animated footage depicts a futuristic fast-moving machine that looks straight out of Star Wars, detects missiles that are fired at and responds almost via telepathy.
The same technology that enables Google’s self-driving cars, which began 10 years ago as a DARPA experiment, could enable differently designed vehicles to react not just to changes in the road ahead but to predict rapidly incoming ordnance.
A bit of robot history: In 2005, a team from Stanford University competing in the second DARPA-sponsored Grand Challenge event developed a car that knew where it was thanks to a continuous relay between its onboard computer and a network of satellites and understood where it was going thanks to the same sort of SICK LMS laser range finders. These were mounted on top of the car to constantly scan the horizon for obstacles and aberrations in the road.
The laser actually acts more like sonar than it does like a pair of eyes. The beam bounces off objects and the bounce rate indicates distance and depth, easily computable signals. Unfortunately, Stanford’s lasers could only project 20 meters ahead. That meant that if the vehicle was to travel at a reasonable rate of speed (35 miles per hour, per DARPA’s guidelines) and it encountered a pitch in the road, the lasers would look upward before returning to their normal trajectory. Suddenly, the terrain would be full of non-rendered space taking the form of large swaths of blackness. The vehicle, obeying its programming, would overcompensate and swerve wildly to avoid hitting what it perceived to be an obstacle—but that was simply area the lasers had not scanned--and dive off the road into the bushes. This was no way to win a race.
Artificial intelligence expert Sebastian Thrun, the team’s leader, understood that he had to get the car to recognize aspects of the road ahead through visual signals, a camera feed. But machine vision remains one of the most difficult aspects of robotics and artificial intelligence. There were two avenues: label every pixel in every frame that the camera was picking up as either road or not road (a labor-intensive route taken by the Carnegie Mellon team) or create an algorithm that could predict where the road would be on the basis of a smaller amount of camera data. But if they took this route then the car would be doing less seeing, more inferring.
The team looked at the digitized samples of roads that the lasers had detected and used those samples to train an algorithm to project those conditions onto future terrain. They matched momentary sensor input with a Markov model for the algorithm. The trick worked. The machine became very good at guessing what the road ahead would be like on the basis of the road it had experienced. The Stanford team completed the DARPA 2005 Grand Challenge race in six hours and 53 minutes, barely nudging out the Carnegie Mellon team for first place and making history.
The trick to getting a car to not only avoid but dodge and weave rockets as if guided by telepathy lies not just in designing a with super shocks and building more sensitive sensors but in writing algorithms that can receive act on sensed data as quickly as a living thing.
NEXT STORY: The FCC Website Will Likely Crash on Wednesday