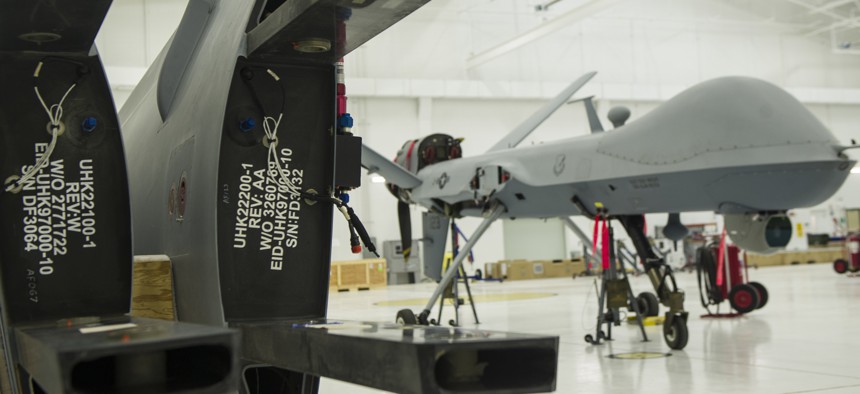
An MQ-9 Reaper sits in a hangar prior to having the wings put on at Holloman Air Force Base, Oct. 13, 2015. US AIR FORCE / EMILY KENNEDY
A New AI Learns Through Observation Alone: What That Means for Drone Surveillance
The military spends hundreds of man hours on intelligence collection and image analysis. Drones that could learn about human behavior with less human guidance could cut that time considerably.
A breakthrough will allow machines to learn by observing. This Turing Learning, as its inventors have named it, promises smarter drones that could detect militants engaging in behavior that could endanger troops, like planting roadside bombs.
Still in its infancy, the new machine learning technique is named for British mathematician Alan Turing, whose famous test challenges artificial intelligences to fool a human into thinking he or she is conversing with another human. In Turing learning a program dubbed the “classifier” tries to learn about a system designed to fool it.
In certain ways, Turing Learning resembles many existing machine-learning systems. As the classifier watches events unfold, it tries to discern patterns of behavior. In their experiment, the researchers used swarming robots. But you could replace swarming robots with any other group displaying some behavior that you want the classifier to learn about: a pack of wolves circling a wounded animal, shoppers taking items from store shelves to a cash register, or an insurgent burying an IED on the side of the road. It’s the classifier’s job to learn how to distinguish between someone just digging a hole and someone else burying a bomb.
A traditional object-classification program is driven by “rewards.” As a self-driving car gets better and better at distinguishing types of obstacles and other features, it receives a reinforcement signal, which tells the program to keep doing that sort of thing.
Turing Learning works a bit differently. It adds another program to the mix: a modeler, which feeds false information to the classifier. The modeler pretends to be the thing being observed — in the experiment, a swarm of hockey-puck robots. If the modeler can convince the classifier that its counterfeit data is real, it receives a reinforcement signal.
With the modeler rewarded for fooling the classifier, and the classifier rewarded for avoiding being fooled, the two programs race to correctly learn the behavior of the objects under observation. That’s what allows the system to learn with much less direct human input.
“Turing Learning thus optimizes models for producing behaviors that are seemingly genuine, in other words, indistinguishable from the behavior of interest. This is in contrast to other system identification methods, which optimize models for producing behavior that is as similar as possible to the behavior of interest,” the researchers write in their paper, published last week in the journal Swarm Intelligence.
“Turing Learning could be applied for the detection of suspicious behavior,” said one of the paper’s authors, Roderich Gross of the University of Sheffield’s Department of Automatic Control and Systems Engineering. But that doesn’t mean it’s ready for prime time. “It may lead to false-positives, for example, if a person with no bad intention happens to behave in a very unusual way, an alarm may trigger.”
Teaching the system to differentiate between benign and suspicious behavior depends on feeding the classifier enough data on regular behavior, thus establishing a strong baseline. Gross described teaching the system to spot shoplifters at the supermarket.
“Ideally, you have an archive of data taken from vast amounts of people that you know were trustworthy,” he said. “This could be, for example, the motion trajectories of shoppers on days where no items went missing. You can then use Turing Learning to automatically build models of shoppers (that are not thieves). Simultaneously, Turing Learning will produce classifiers (interrogators) which, given a sample of data, can tell whether a person is likely to be thief. These classifiers could then be used in security applications.”
What are the possible implications for the military? Every day, analysts sit through hours of surveillance footage in places like Creech Air Force Base in Nevada, watching human behavior, looking for patterns of life, seeking to determine who might pose a threat.
It’s tedious work, and there’s plenty of it. The U.S. Air Force was processing upwards of 1,500 hours of drone footage collected from Predators and Reapers everyday in 2011, according to the New York Times. “Hundreds” of hours of observation and analysis go into preparing for a strike, Air Force Col. Jim Cluff told reporters visiting Creech last year.
Steven K. Rogers, the senior scientist for automatic target recognition and sensor fusion at the Air Force Research Laboratory, described what that looks like at last summer’s GEOINT conference.
“I have young airmen, analysts. They are ordered: ‘You stare at that screen. You call out anything you see. If you need to turn your eyes for any reason — you need to sneeze? — you need to ask permission, because someone else has to come put their eyes on that screen.’ The point I’m driving home to you is that the state of the art in our business is people,” he said. “The diversity of these tasks means that quite often, we throw together ad hoc combinations of sensors and people and resources to find information we need.”
It’s one reason why the military last year resorted to hiring contractors to do more of the analysis work, which can cost more money, though the Pentagon has not said how much more they are paying for contractors flying ISR drone missions. Teaching machines to fly those missions themselves would free operators to do more.