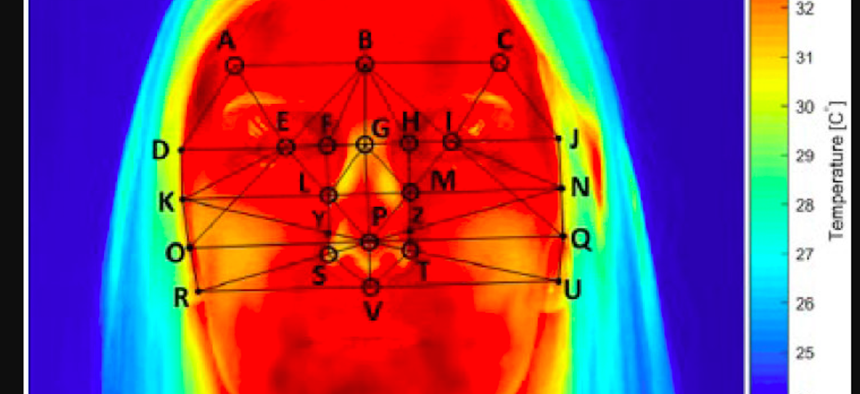
An example of an attempt to apply facial recognition techniques to thermal images. Adrian Cornelius Marinescu See all articles by this author Search Google Scholar for this author , Sarah Sharples, Alastair Campbell Ritchie, Tomas Sánchez López, Michael M University of Nottingham
US Army Figures Out How To Do Facial Recognition in the Dark
New method uses machine learning to extrapolate features from thermal images.
It’s no mean feat for a computer to identify an individual’s face in daylight. The process involves precisely measuring a photograph — eye size, distance from nose to mouth, etc. — adjusting the distances for three dimensions, and searching a database for a match. But to do it at night, when all you have is far lower-resolution thermal images, the Army Research Lab used a technique that allows software to mimic the human brain.
Our brains “see” by extrapolating a picture from a relatively small amount of sensory data, filtered through the eye. The brain uses several times more neuronal mass to construct images from visual data than the eye does collecting the data.
The Army researchers saw a parallel with thermal images. Such images show what parts of the face are hotter and cooler, but generally contain fewer data points than a comparable optical image from a camera, making it hard to pick out distinct features. So they set up a convolutional neural network, or CNN, a deep-learning method that uses specific nodes similar to the brain’s, and set it to infer faces from limited data.
The method that the researchers use breaks a thermal picture of a face into specific regions and then compares them to an optical image of the same face. The network estimates where key features are in the thermal image in relation to the conventional image. The network’s final product is something like a police sketch — not a perfect match, but with enough overlap in key points to make a high-certainty match.
In a paper published by the IEEE Winter Conference on Applications of Computer Vision, the researchers write, “We were able to produce highly discriminative representations. Despite the fact that the synthesized imagery does not produce a photo-realistic texture, the verification performance achieved was better than both baseline and recent approaches when matching the synthesized faces with visible face.”
On Tuesday, the Lab released a statement quoting researcher Benjamin S. Riggan, who said, “When using thermal cameras to capture facial imagery, the main challenge is that the captured thermal image must be matched against a watch list or gallery that only contains conventional visible imagery from known persons of interest...Therefore, the problem becomes what is referred to as cross-spectrum, or heterogeneous, face recognition. In this case, facial probe imagery acquired in one modality is matched against a gallery database acquired using a different imaging modality.”