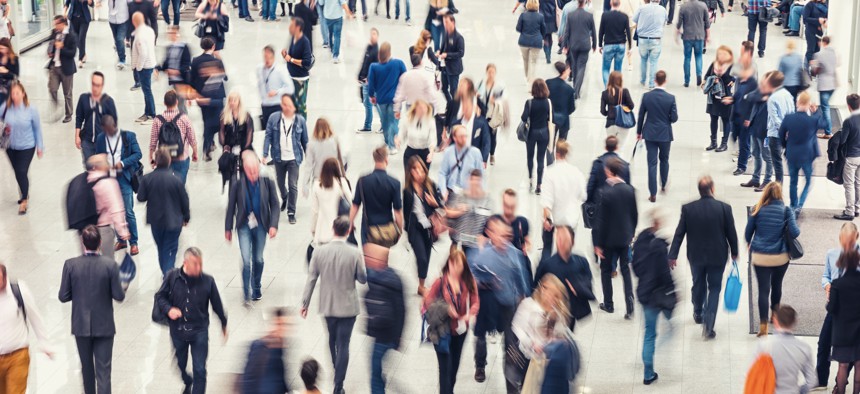
r.classen / Shutterstock
AI Is Already Keeping Tabs on 1 Million Clearance Holders
Trusted Workforce 2.0 tools regularly scan a set of data sources for anomalies in the behaviors of more than a million federal and private workers.
The security clearance process is broken—a fact widely accepted by stakeholders in the public and private sector, legislative and executive branches of government, Democrats and Republicans. As federal leaders work on the largest process overhaul in half a century, artificial intelligence will play a key role.
In February, officials unveiled plans for Trusted Workforce 2.0, a framework that would shift suitability and security determinations from a one-time investigation followed by reassessments every five to 10 years, to an ongoing process that uses technology and private sector partners and data.
The first step in moving away from the old process is getting rid of all the paper, according to Terry Carpenter, the program executive officer for the National Background Investigation Service, the office overseeing the technical overhaul of the investigations process.
“The days of you filling out some form—online or in paper—submitting this form; having people go through that form; analyze your responses; decide which investigator to send out there to meet with your parents, your friends, your neighbors, who may all be in different states; to write up reports and assemble a package that grows as we do the investigation and come back to somebody to adjudicate the recommendation to say, ‘should this person get a clearance or not based on policy?’ We can’t do that anymore—that’s paper,” Carpenter said Thursday during the Government Analytics Breakfast Forum hosted by Johns Hopkins University and REI Systems.
Related: The Security Clearance Process Is About to Get Its Biggest Overhaul in 50 Years
Related: Security Clearance Delays Are Hurting the Pentagon’s Tech Workforce
Related: The US Military Is Creating the Future of Employee Monitoring
Before instituting the Trusted Workforce 2.0 framework, adjudicators would have to physically travel to interview people on every topic covered by the clearance investigation. Under the new guidance, investigators have the option to use other means to speed the process.
In October, Carpenter’s team rolled out a tool to digitize the front end of the security clearance process: filling out paper forms like the SF-86.
The NBIS team developed a digital form that operates similarly to modern tax preparation software. Users are guided through a set of questions and document requests that ensure the data is entered in a structured format.
Another tool currently in development uses artificial intelligence to pull investigatees’ data from multiple sources, including public information, private data sources like credit reports and government data, either through interagency partners or data collected by investigators. The AI tool will gather and sort that data and present it to adjudicators in a structured, interactive format.
“We have a complete list of how the algorithm got to the recommendation. We can click on any piece of data in that decision-making chain and see it. And that gets packaged up as part of the supporting evidence for why decisions were made,” Carpenter said.
With digitized data sources and AI helping pull and sort the information, the investigative process can move toward a “continuous evaluation” model, in which clearance holders are regularly reassessed based on new information, rather than on an arbitrary, decade-long cycle.
“We already have 1.1 million names in as part of the initiative and it just goes through the data sources on some reoccurring schedule set by the business rules and if an anomaly comes up, then we look at the anomaly and decide what to do with it,” Carpenter said. “Maybe I got a speeding ticket last night and it popped up on the data source,” he offered as a hypothetical.
Carpenter said the team hopes soon to be able to pilot the system end-to-end with the lowest level suitability determinations. If that pilot succeeds, the team can add more data streams and capabilities in order to handle more sensitive assessments.
All this will be possible because the team looked at the problem as a whole, rather than just finding cool tools to apply to parts of the problem, Carpenter said.
“We moved the NBIS architecture to an enterprise data broker concept and we moved the data sources to one place,” he explained. “This gave us the ability to focus on control of that data. I don’t have to own it all—it doesn’t have to sit on my servers. … But we need to know that we have the connection, we have the ability to pull it when we need it, we know what the data is—what it represents—and we know the policies and agreements—the laws—that surround the use of that data.”
“We couldn’t rush to the quick answer,” he added. “We had to do some of these critical, I call them ‘hygiene steps.’”
No matter what, it will be up to humans to make the final decisions about suitability and trust, Carpenter said.
“No decision will be made by a machine. We will augment decision- making,” he said. “Instead of them spending weeks and months trying to find needles in a haystack, we’re using artificial intelligence and machine learning to present those needles, in a neat form with all the relevant information to how we found it for the human to make that final determination.”