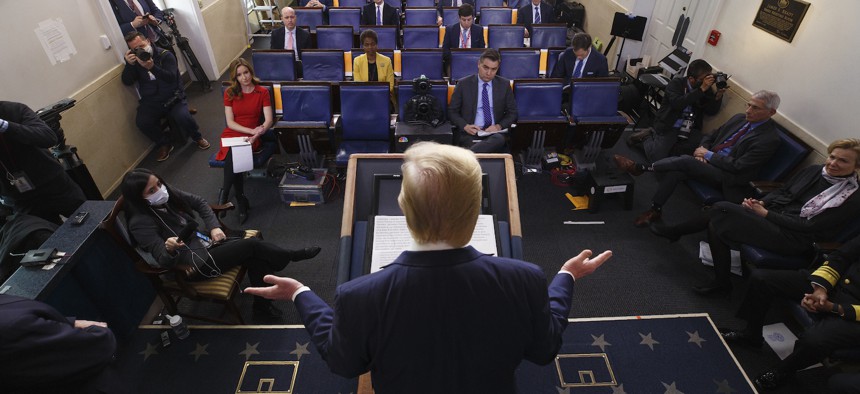
President Donald Trump speaks during a coronavirus task force briefing at the White House, Friday, April 10, 2020, in Washington. AP Photo/Evan Vucci
SPECIAL REPORT: The Problem With Coronavirus Models Is How We Talk About Them
Despite what political leaders want them to say, COVID-19 models will become less predictive exactly when we need them most.
In the continuing drama of America’s coronavirus response, statistical models play the role of Hamlet’s father: foreboding, influencing the action on the stage but unable to speak for themselves.
Some health leaders have said that various COVID-19 models’ worst projections — such as the Imperial College London model that predicted that 500,000 Britons would die — were overly dire. That skepticism about what the models truly say will play a growing role in the discussion about when and how to “re-open” the country. But the idea that the worst of the pandemic is behind us — and that the models prove it — is misleading at best and dangerous at worst, say experts.
Right now, the world is eager for news that the crisis is abating and that economic and social life can “return to normal.” Largely as a result of massive social distancing efforts, new infections in New York and other hotspots are going down. Some, like Andrew McCarthy at the National Review, feel like they’ve seen enough to toss the modelers out on their rears. “There is no shortage of government spin, regurgitated by media commentators, assuring us that the drastic reductions in the projections over just a few days powerfully illustrate how well social distancing and the substantial shuttering of the economy is working. Nonsense,” McCarthy writes.
What the models are, in fact, is poorly represented by the policy makers touting them. The vast majority of them aren’t built to project infection rates out beyond a few weeks, so they don’t tell us anything about the resurgences that may come. Some of the most influential models, such as the University of Pennsylvania’s COVID-19 Hospital Impact Model for Epidemics, or CHIME, were designed to help hospital managers stock up for worst-case scenarios. Yet in the Washington, D.C., mayor’s office, CHIME is the model of choice.
CHIME is an SIR model, which stands for "susceptible, infected, recovered." Such models are hardly perfect and they don’t pretend to be, as Michael Z. Levy, an associate professor in the Department of Biostatistics, Epidemiology & Informatics at the University of Pennsylvania, recently said. You can change the outcome drastically with small changes to various parameters. For it to be of the most use, you need to be seeking the answer to a specific question, like “How many beds will this hospital need in May?”
SIR models assume that “everyone is bumping into everyone else like perfect spheres in a vacuum,” Levy said. “We didn’t act like that even before social distancing.”
Such models are “fine for short-term forecasts, but it becomes problematic when you start to ask different questions,” he said. What will happen if social distancing is implemented in some places but not others? What happens if infections go down but testing is still inadequate? What changes if therapeutic drugs are available only in some places?
The Institute for Health Metrics and Evaluation, or IHME, model used by the White House is a more general public-health model. It predicted peak hospital resource use during the second week in April, along with ICU and hospital overcapacity. But it showed those outcomes could be mitigated through social distancing measures. When most people talk about “flattening the curve,” they’re referring to this model.
But the model’s creators admit to a big limitation that doesn’t make it into the White House briefings. It was originally designed only to track infection rates and hospital use through June. And while its creators at the University of Washington are constantly using new data to improve it, these updates don’t provide high-confidence predictions about the fall or next year because the number of variables is growing as new measures are implemented in lots of different ways across varied locations.
Another example: a March 25 article in the New York Times was intended to show the effects of two months of social distancing to “flatten the curve.” But it doesn’t extend past October. Wesley Pegden, an associate professor in the Department of Mathematical Sciences at Carnegie Mellon University, recently took to Medium to point out that two months of lockdown won’t end the pandemic, and people who say otherwise are mistaken or lying. In fact, a two-month lock down that isn’t followed by a uniform strategy to manage new infections without overwhelming the health system leads to a fall resurgence that eclipses the spring “peak.”
“Two months of mitigations have not improved the outcome of the epidemic in this model, it has just delayed its terrible effects,” Pegden wrote. “In fact, because of the role of weather in the model presented in the [New York Times] article, two months of mitigations actually results in 50% more infections and deaths than two weeks of mitigations, since it pushes the peak of the epidemic to the winter instead of the summer, whose warmer months this model assumes causes lower transmission rates...No model whose purpose is to study the overall benefits of mitigations should end at a time-point before a steady-state is reached.”
In a conversation with Defense One, Pegden said that the messaging around these models has left the public ill-informed. “People have not been given enough sense of the uncertainty. They have not been given a sense of how a lockdown will not fix the problem.”
When he tells people this, he finds them “surprised and disturbed that a two-month lockdown was not going to fix the problem,” he said.
Pegden’s concerns are much more in line with a model produced by Neil Ferguson of Imperial College London. It predicts resurgent infection spikes until a vaccine becomes widely available in a year or so.
Even the most dire projections remain a possibility despite the recent slump in new cases, Ferguson said.
1/4 - I think it would be helpful if I cleared up some confusion that has emerged in recent days. Some have interpreted my evidence to a UK parliamentary committee as indicating we have substantially revised our assessments of the potential mortality impact of COVID-19.
— neil_ferguson (@neil_ferguson) March 26, 2020
Pegden says that a lockdown from March to May could wind up making the situation much worse later precisely because policymakers overpromised on its effects. A lockdown only buys you time, time to get nationwide testing in order, ventilators to more places, and hospitals ready for a coming surge in patients. In the United States, that hasn’t happened to the extent it needs to.
“It is not true, a priori, that every transmission you avoid today is saving lives,” says Pegden. “You can save lives by having more transmissions among more people in the short term.”
He acknowledges that the idea of exposing more people to the virus is counterintuitive, but, he says, “It’s not always the case that the most conservative thing you can do will save the most lives. If transmission levels revert to normal over the next year...then it would turn out that being locked down was a mistake.”
The Models We Need Now
It’s now up to policymakers to find a coherent way to reopen the country, guided by strategy and not models that will start to fall apart as more and more variables are introduced. A total lockdown until a vaccine arrives is neither politically nor economically viable. The question is how to ease the lockdown without overwhelming the healthcare system. Pegden put out a preprint paper looking at how to do just that. In essence, he says, you want to start lifting restrictions on people who are younger, a population that is less likely to need ICU care. But you don’t want to lift restrictions on all of them since some of them may live with someone in the more vulnerable 60-plus age group.
“Age-targeting has the potential to greatly reduce total mortalities compared with optimum choices for homogeneous measures. At the same time, we see that the best strategies for age-targeting are sensitive to the fraction of the younger population which can be released. In general, if too few people are released initially, a second wave occurs when transmission levels return to normal,” they write.
A visual representation of the effects of relaxing social distancing rules for some individuals under the age of 50 versus the entire U.S. population, from “Modeling strict age-targeted mitigation strategies for COVID-19”
Policy-makers should also begin to prepare for the illness to start having a much worse effect on people with lower incomes. Reports show that they are more likely to have underlying health conditions and are less likely to be able to work remotely or to maintain social distance, said Levy. That diversity of health outcomes is already occurring to some extent, but it’s likely to get worse. A new visualization tool shows which areas are going to be more affected based on the demographics of the population.
Levy, in his lecture, pointed out that trying to tweak models to reflect the arrival of anti-COVID drugs won’t much improve them, since there are still too many variables to control for. “To really affect transmission dynamics,” he said, a potential drug “would need to affect these other parameters. You would need an over-the-counter-cold-sniffy-nose-and-COVID drug.”
One thing we probably should begin to model for now is mutation, since that’s going to affect the efficacy of drugs, vaccines, and other measures later on. That's going to be more useful than trying to forecast infection and recovery among a population in an environment where that will become more difficult.
A recent paper by researchers at Carnegie Mellon University offers an interesting and useful take on precisely that very hard problem.
Predicting when a virus mutates into another virus is more challenging than mapping the spread of a contagion among a group. But looking at how information spreads and mutates online in social networks can provide some insight into how viruses spread and mutate in the real world.
“There is some similarity from an evolution perspective via the mechanism of natural selection. A virus may go through a number of mutations (mostly a random process). However, among a large number of these, the mutation that leads to highest infectiousness will be ‘naturally selected’ i.e., it will be the one that we will see most commonly. For information it is also similar,” Osman Yağan, an associate research professor at Carnegie Mellon’s Department of Electrical and Computer Engineering, told Defense One in an email. “Several people can attempt to modify a piece of information to make it more attractive. It will be the audience who will then ‘select’ the most appealing one and that particular version of the information will be much more prevalent (say in Twitter) than others.”
Yağan says that the research could help epidemiologists better prepare in case the virus goes through a rapid mutation process, as well as to understand the effects of protective measures in a new way. “We believe that there is some similarity between ‘a virus mutating and becoming less easy to infect individuals’ and ‘people being careful about social-distancing and thus becoming less easy to get infected.’ The analogy here is strengthened by the fact that those who do not obey social-distancing are more likely to infect each other (a la carrying a second-strain of the virus) than those who are obeying the guidelines.”
The work is partially funded by the U.S. Army. Edward Palazzolo, program manager for the Social and Cognitive Networks Program at the Army Research Office, told Defense One that it's his job to bring people together across different disciplines to find new approaches to problems, which is what they did here. “We’re always supporting basic research” he said, and it's a long-term effort. They began funding Yağan’s work in 2017.
Speaking in a personal capacity and not as a representative of the U.S. Army, Palazzolo said that he was heartened to see more people learning some of the elementary basics of data science. But he said that contract tracing — a key to other countries’ successful approaches — will be especially difficult in the United States because of the privacy implications of contact-tracing apps.
Palazzolo warned that leaders should avoid treating models like crystal balls. They “should never take a model and say, ‘This is the truth’,” he said.
More importantly, they need to work with scientists to understand statistical terms — for example, what a confidence interval is. (It’s the specific range of numbers the modeler is confident that the value falls within.) “Having the right people on the team will make all the difference.”
Of course, having the right people only matters if you listen to them — lest we rely on models that seem to predict a recovery on the basis of assumptions that are no-longer true, like a tale told by an idiot, full of sound and fury, signifying nothing.