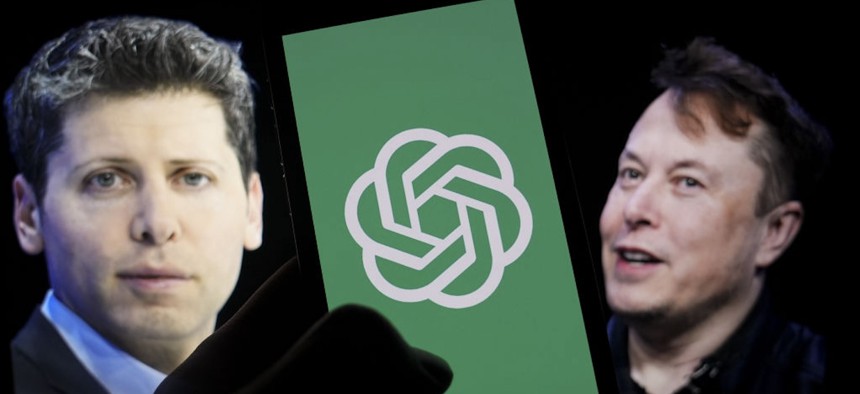
OpenAI's Sam Altman, right, his company's logo, and xAI's Elon Musk Muhammed Selim Korkutata/Anadolu via Getty Images
Is ‘Big AI’ beating 'small AI'—and what does it mean for the military?
Efforts to build giant, power-hungry models may be squeezing out the kind of computing-at-the-edge projects the military actually needs.
The prevailing "bigger-is-better" approach to artificial intelligence—ingest more training data, produce larger models, build bigger data centers—might be undermining the kind of research and development the U.S. military actually needs now and in the future.
That’s the argument in "Hype, Sustainability, and the Price of the Bigger-is-Better Paradigm in AI," a new paper that scrutinizes common assumptions driving AI research. Its authors demonstrate that the performance of larger models doesn’t necessarily justify the vastly increased resources needed to build and power them. They also argue that concentrating AI efforts in a relative handful of big tech companies adds geopolitical risks.
Broadly speaking, the Defense Department is pursuing AI along two tracks: large models that require enormous computational resources, and smaller, on-platform AI that can function disconnected from the internet. In some ways, the study validates the second approach. But, the authors note, future research in “small AI” could be limited due to growing influence of large AI providers.
Where does the idea that bigger is better, at least in AI, come from? In their paper, Gaël Varoquaux of Université Paris-Saclay, Alexandra Sasha Luccioni of the Quebec AI Institute, and Meredith Whittaker of the Signal Foundation trace it to a 2012 paper by University of Toronto professor Alex Krizhevsk, who argued big data and large-scale neural networks offered much better results for image classification than smaller ones. This idea was borne out by other researchers, and has since become a staple of the way large companies are approaching AI.
“The consequence of this is both an explosion in investment in large-scale AI models and a concomitant spike in the size of the notable (highly cited) models. Generative AI, whether for images or text, has taken this assumption to a new level, both within the AI research discipline and as a component of the popular ‘bigger-is-better’ narrative surrounding AI,” they write.
The authors gather evidence to show that benefits of scaling up AI models diminish rapidly compared to the increased computational demands. For instance, the environmental cost—measured in energy consumption—rises significantly faster than the improvement in model performance, making large-scale AI not especially efficient. That’s lost on a lot of big AI’s richest and most well-known boosters like former Google chairman Eric Schmidt, who argued last week that businesses and governments should continue to pursue energy-intensive large AI models regardless of the energy cost because “we're not going to hit the climate goals anyway.”
The military can’t take such a cavalier approach to the massive energy costs of AI. The Defense Department views climate change as a national security concern but, more immediately, also views energy efficiency as a key military objective for future operations.
What’s worse, the bigger-is-better conventional wisdom AI research is narrowing and losing diversity, they write.““The bigger-is-better norm is also self-reinforcing, shaping the AI research field by informing what kinds of research is incentivized.”
That means that researchers will increasingly ignore areas where smaller models could make a big difference, in fields like healthcare and education.
Although the authors don’t address it in their paper, that narrowing effect has ramifications for the military’s own development of AI. Smaller models could also make a big difference in places where computer resources are scarce and connectivity is intermittent, sparse, or even non-existent. That could apply to everything from autonomous drones operating in environments saturated with adversary electromagnetic warfare effects to small bases in forward locations where energy is scarce and connectivity is weak.
The rapid evolution of weapons and tactics means that more and more operators close to the edge of combat will have to invent or modify their own gear and weapons. Operators at forward bases might face a lot of situations where they may have good use for an AI model that runs on a relatively small corpus of data and doesn’t require a massive server farm or lots of GPUs to work. These might include applications that analyze drone- or satellite-image data for specific types of vehicles , parsing the specific electromagnetic weapons signatures they are encountering, or even just understanding local economic, weather, population or consumer data to plan more effective and safe operations in dense urban settings. But if the AI research field prioritizes expertise in big AI over small, that could mean less scholarship and fewer experts to train operators in how to make their own small AI models well.
The growing trend toward big AI has another geopolitical implication, a concentration of power. Only a few companies possess the resources to build and deploy massive models. “The concentrated private industry power over AI creates a small, and financially incentivized segment of AI decision makers. We should consider how such concentrated power with agency over centralized AI could shape society under more authoritarian conditions,” they write.
One obvious example of the threat that poses is Elon Musk, one of the world’s richest defense contractors and, through SpaceX, a key supplier of space access and satellite communications to the Pentagon. Musk also has close financial ties to Saudi Arabia and has used his large and expensive social media presence to boost posts and content linked to Russian disinformation operations. Musk is also emerging as one of the key financial players in the development of future AI.
Whittaker and her fellow authors are among a small but growing number of AI-focused researchers who point out the risks posed by the prevalence of the bigger-is-better school of AI. A separate paper, published in September by a group of researchers at Berkeley also notes “It is exceedingly common for smaller, more task-focused models to perform better than large, broad-purpose models on specific downstream tasks.”
A new class of innovative AI practitioners are also highlighting the degree to which the conversation around big AI is drowning out approaches that could be more useful for specific groups.
Pete Warden, CEO of AI startup Useful Sensors, is one of them. Warden’s work focuses on embedding intelligence into devices or computers. He says that the industry and academic obsession with larger AI is missing what most people actually want from the AI they interact with.
“Academic benchmarks have diverged from real-world requirements,” Warden said. “For example a lot of customers just want to be able to retrieve results from existing information (like user manuals) rather than generating new text in response to questions, but that isn't seen as interesting by researchers.” Retrieval-Augmented Generation, he said, is an academic hobby horse. But customer applications don't need that level of complexity,” he told Defense One.
“For a lot of realistic problems, like drone tracking for example, the underlying models are now good enough and the real challenge is integrating them into larger systems. We don't need any more computer vision breakthroughs or new model architectures. We just need better data that reflects the actual problems in deployment and a way to fit the models onto hardware.”
Drew Breunig ran data science and strategic client projects at PlaceIQ, which is now part of Precisely. In September he wrote a post on how many peoples’ high expectations of generative AI, the quintessential example of large AI models, are unlikely to be met. When those realizations settle in, that could lead to a broader discussion about different potential paths for AI development.
Breunig told Defense One: “The capability of our existing models greatly outpace the [user interface] and frameworks we've built to deliver their intelligence for nearly all the real world problems they solve.”
He breaks AI into three groups. “Gods,” which he defines as “Superintelligence AGI stuff. Replacement for humans doing lots of different things, unsupervised.”
Beneath them are “interns” which he describes as “Copilots. Domain-specific applications that help experts with busy and tedious work, doing things an intern might. Interns are supervised by experts, so your tolerance for hallucinations is high. The programmer, writer, or whomever spots the mistakes when they occur and moves on.”
Finally, the most local form of AI is “Cogs,” defined as “Models that have been tuned to do one job, with very low tolerance for errors, working unsupervised within applications or pipelines. This is by far the dominant use case I am seeing in the enterprise. All the big platforms (AWS, Azure, Databricks, etc) have pivoted to helping companies load their proprietary data to tune open models to do one little thing well.”
Even though generative AI pilots get a lot more attention the military is exploring all three of them through programs that provide decision assistance for target identification, i.e. Project Maven, and other decision assistance efforts,” and “cog” efforts such as visual recognition of instrument indicators in helicopter cockpits.
Because such needs will only grow, it’s important for the military that the future of AI research be broad enough to continue to support all three areas.
“Pete and I see eye to eye on this,” said Breunig. “When Pete says the academics' research cases are out of step with the practical reality of actually building with AI, it's because a lot of their focus is on attaining gods not the boring job of building cogs.The cool thing about focusing on cogs is you can do so much with little models! A tiny model tuned for one thing can outperform a giant general model at the same task. And it's faster, cheaper, etc.”